The manuscript entitled “SICK through the SemEval glasses. Lesson learned from the evaluation of compositional distributional semantic models on full sentences through semantic relatedness and textual entailment” by Luisa Bentivogli, Raffaella Bernardi, Marco Marelli, Stefano Menini, Marco Baroni, Roberto Zamparelli has been published in the Language Resources Evaluation (LRE) journal.
Abstract.
This paper is an extended description of SemEval-2014 Task 1, the task on the evaluation of Compositional Distributional Semantics Models on full sentences. Systems participating in the task were presented with pairs of sentences and were evaluated on their ability to predict human judgments on (1) semantic relatedness and (2) entailment. Training and testing data were subsets of the SICK (Sentences Involving Compositional Knowledge) data set. SICK was developed with the aim of providing a proper benchmark to evaluate compositional semantic systems, though task participation was open to systems based on any approach. Taking advantage of the SemEval experience, in this paper we analyze the SICK data set, in order to evaluate the extent to which it meets its design goal and to shed light on the linguistic phenomena that are still challenging for state-of-the-art computational semantic systems. Qualitative and quantitative error analyses show that many systems are quite sensitive to changes in the proportion of sentence pair types, and degrade in the presence of additional lexico-syntactic complexities which do not affect human judgements. More compositional systems seem to perform better when the task proportions are changed, but the effect needs further confirmation.
Keywords.
Compositionality – Computational semantics – Distributional semantics models.
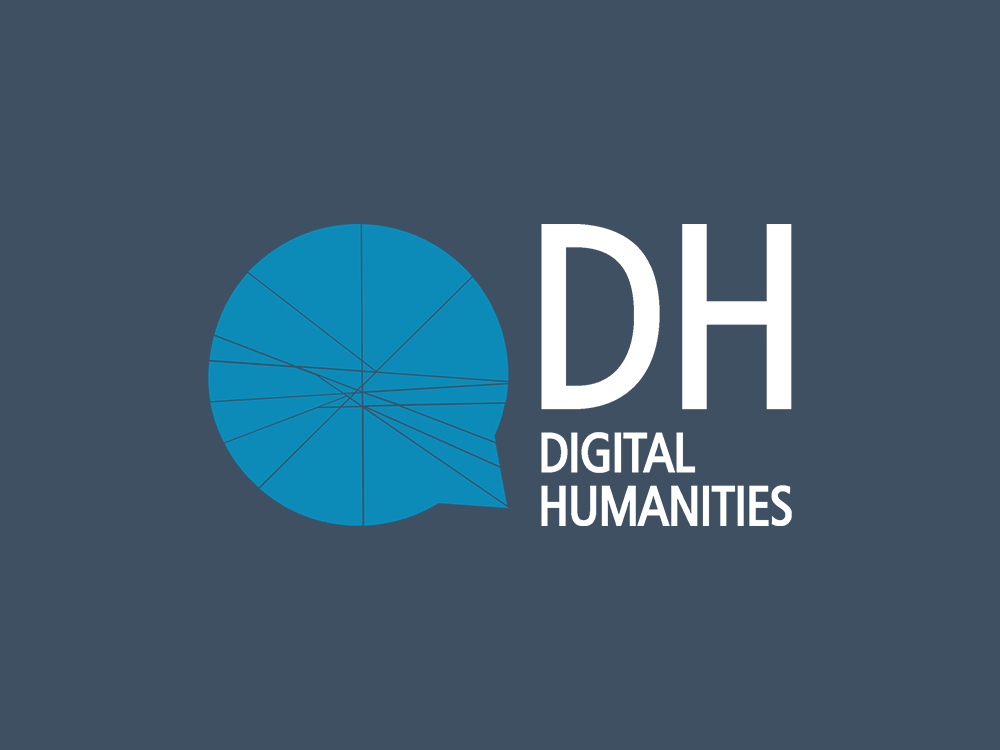
Website: