The short paper “Topic-Based Agreement and Disagreement in US Electoral Manifestos” by Stefano Menini, Federico Nanni, Simone Paolo Ponzetto and Sara Tonelli has been accepted at the 2017 Conference on Empirical Methods on Natural Language Processing (EMNLP2017).
This work is a joint collaboration with the Data and Web Science Group at the Universität Mannheim.
Abstract:
In this work, we present a topic-based analysis of agreement and disagreement in political manifestos, which relies on a new method for topic detection based on key concept clustering. Our approach outperforms both standard solutions like LDA and a state-of-the-art graph-based method, and provides first promising results for this new task in computational social science.
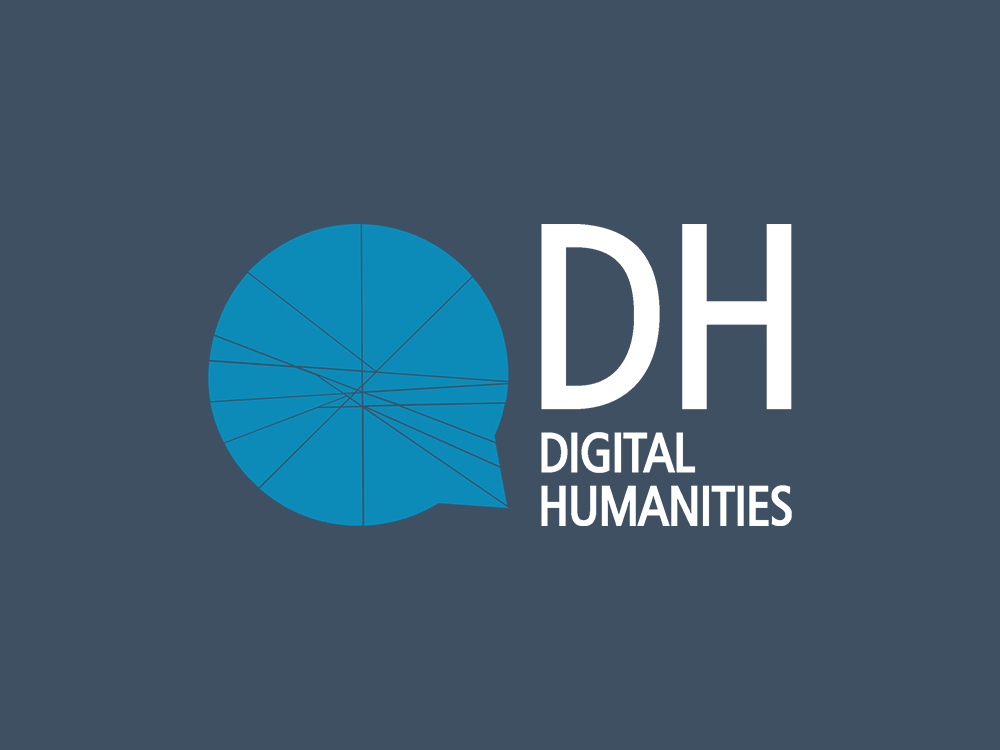