Our group has two accepted papers at EMNLP 2021!
Title “Agreeing to Disagree: Annotating Offensive Language Datasets with Annotators’ Disagreement” authored by Elisa Leonardelli, Stefano Menini, Alessio Palmero Aprosio, Marco Guerini and Sara Tonelli. Long paper accepted at EMNLP2021 main conference.
Since state-of-the-art approaches to offensive language detection rely on supervised learning, it is crucial to quickly adapt them to the continuously evolving scenario of social media. While several approaches have been proposed to tackle the problem from an algorithmic perspective, so to reduce the need for annotated data, less attention has been paid to the quality of these data. Following a trend that has emerged recently, we focus on the level of agreement among annotators while selecting data to create offensive language datasets, a task involving a high level of subjectivity. Our study comprises the creation of three novel datasets of English tweets covering different topics and having five crowd-sourced judgments each. We also present an extensive set of experiments showing that selecting training and test data according to different levels of an- notators’ agreement has a strong effect on classifiers performance and robustness. Our findings are further validated in cross-domain experiments and studied using a popular benchmark dataset. We show that such hard cases, where low agreement is present, are not necessarily due to poor-quality annotation and we advocate for a higher presence of ambiguous cases in future datasets, particularly in test sets, to better account for the different points of view expressed online.
Link to preprint: https://arxiv.org/pdf/2109.13563.pdf
Link to dataset: https://github.com/dhfbk/annotators-agreement-dataset
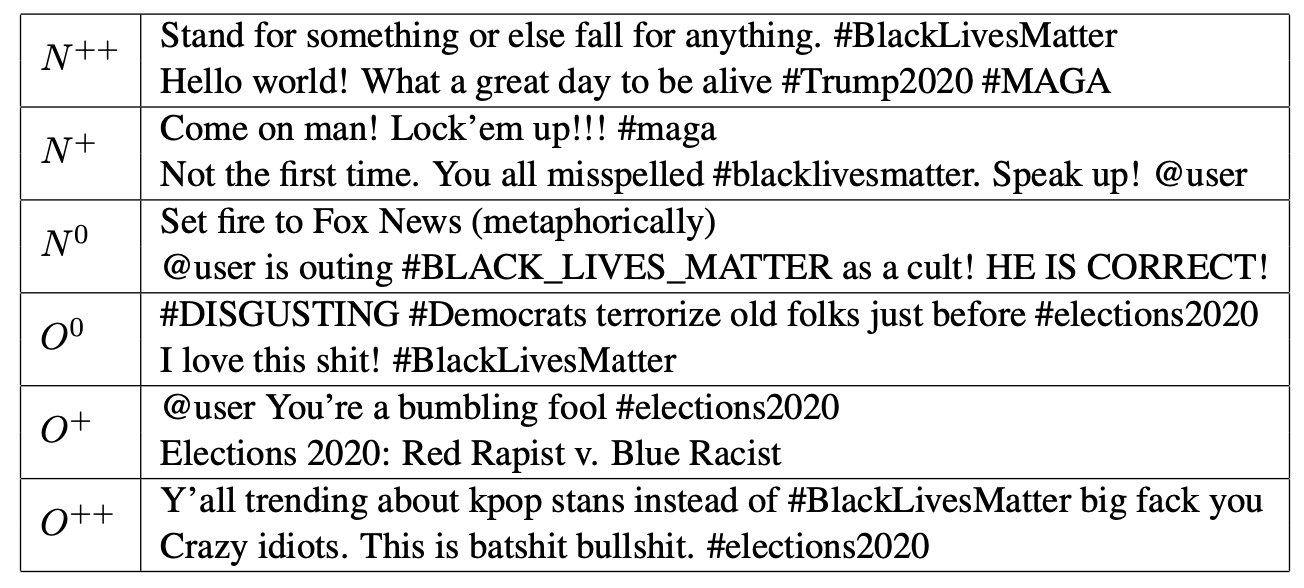
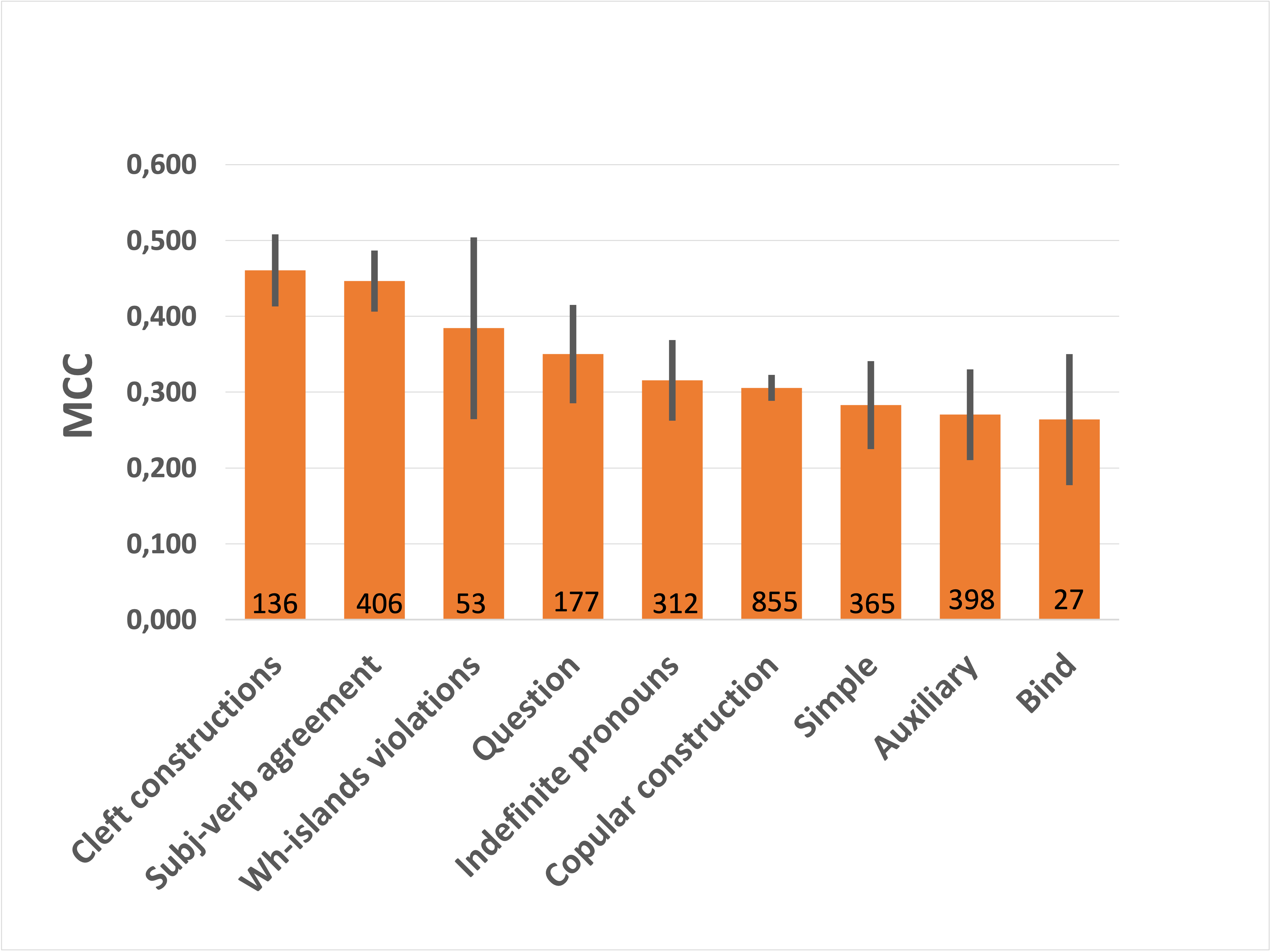
Title: “Monolingual and Cross-Lingual Acceptability Judgments with the Italian CoLA corpus” authored by Daniela Trotta, Raffaele Guarasci, Elisa Leonardelli and Sara Tonelli, accepted in Findings of EMNLP2021
The development of automated approaches to linguistic acceptability has been greatly fostered by the availability of the English CoLA corpus, which has also been included in the widely used GLUE benchmark. However, this kind of research for languages other than English, as well as the analysis of cross-lingual approaches, has been hindered by the lack of resources with a comparable size in other languages. We have therefore developed the Ita-CoLA corpus, containing almost 10,000 sentences with acceptability judgments, which has been created following the same approach and the same steps as the English one. In this paper we describe the corpus creation, we detail its content, and we present the first experiments on this new resource. We compare in-domain and out-of-domain classification, and perform a specific evaluation of nine linguistic phenomena. We also present the first cross-lingual experiments, aimed at assessing whether multilingual transformer- based approaches can benefit from using sentences in two languages during fine-tuning.
Link to preprint: https://arxiv.org/abs/2109.12053
Link to dataset: https://github.com/dhfbk/ItaCoLA-dataset