Three papers from our group have been accepted at the 26th International Conference on Computational Linguistics (Coling2016):
Stefano Menini and Sara Tonelli “Agreement and Disagreement: Comparison of points of view in the political domain”
Abstract:
The automated comparison of points of view between two politicians is a very challenging task, due not only to the lack of annotated resources, but also to the different dimensions participating to the definition of agreement and disagreement. In order to shed light on this complex task, we first carry out a pilot study to manually annotate the components involved in detecting agreement and disagreement. Then, based on these findings, we implement different features to capture them automatically via supervised classification. We do not focus on debates in dialogical form, but we rather consider sets of documents, in which politicians may express their position with respect to different topics in an implicit or explicit way, like during an electoral campaign. We create and make available three different datasets, and show that our approach is effective on each of them.
Paramita Mirza and Sara Tonelli “CATENA: CAusal and Temporal relation Extraction from NAtural language texts”
Abstract:
We present CATENA, a sieve-based system to perform temporal and causal relation extraction and classification, exploiting also the interaction between the temporal and the causal model. We evaluate the performance of each sieve, showing that the rule-based, the machine learning and the reasoning components all contribute to achieving state-of-the-art performance on TempEval-3 and TimeBank-Dense data. Although causal relations are much sparser than temporal ones, the architecture and the selected features are mostly suitable to serve both tasks. The effects of the interaction between the temporal and the causal components, although limited, yield promising results and confirm the tight connection between the temporal and the causal dimension of texts.
Paramita Mirza and Sara Tonelli “On the contribution of word embeddings to temporal relation classification”
Abstract:
Temporal relation classification is a challenging task, especially when there are no explicit markers to characterise the relation between temporal entities. This occurs frequently in inter-sentential relations, whose entities are not connected via direct syntactic relations making classification even more difficult. In these cases, resorting to features that focus on the semantic content of the event words may be very beneficial for inferring implicit relations. Specifically, while morpho-syntactic and context features are considered sufficient for classifying event-timex pairs, we believe that exploiting distributional semantic information about event words can benefit supervised classification of other types of pairs. In this work, we assess the impact of using word embeddings as features for event words in classifying temporal relations of event-event and event-DCT pairs.
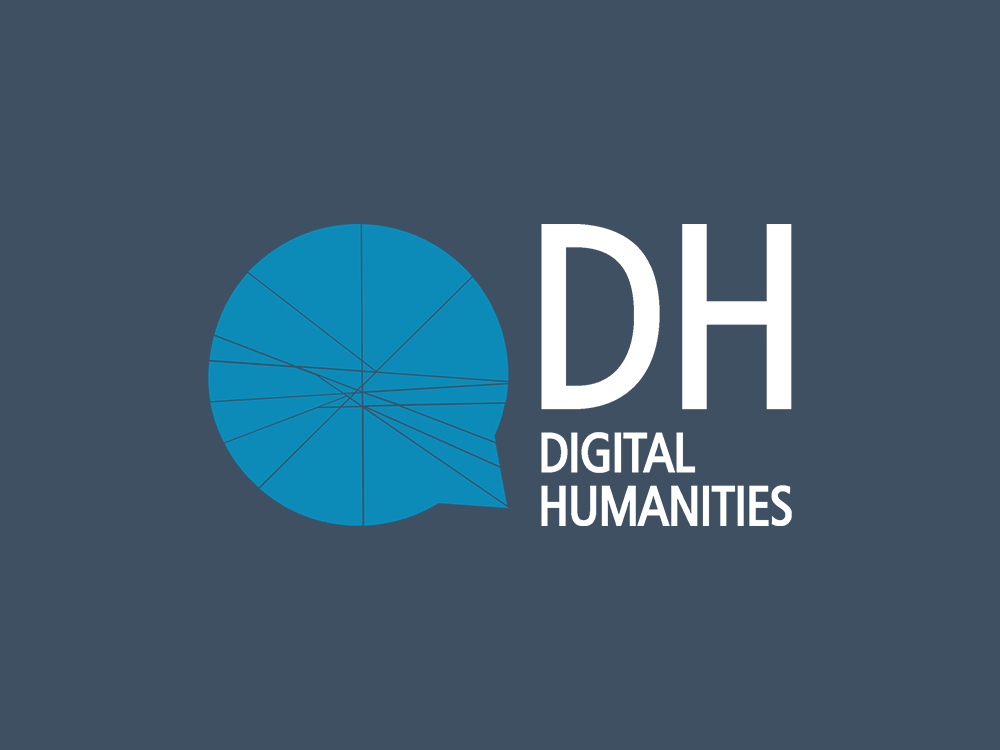
Website: